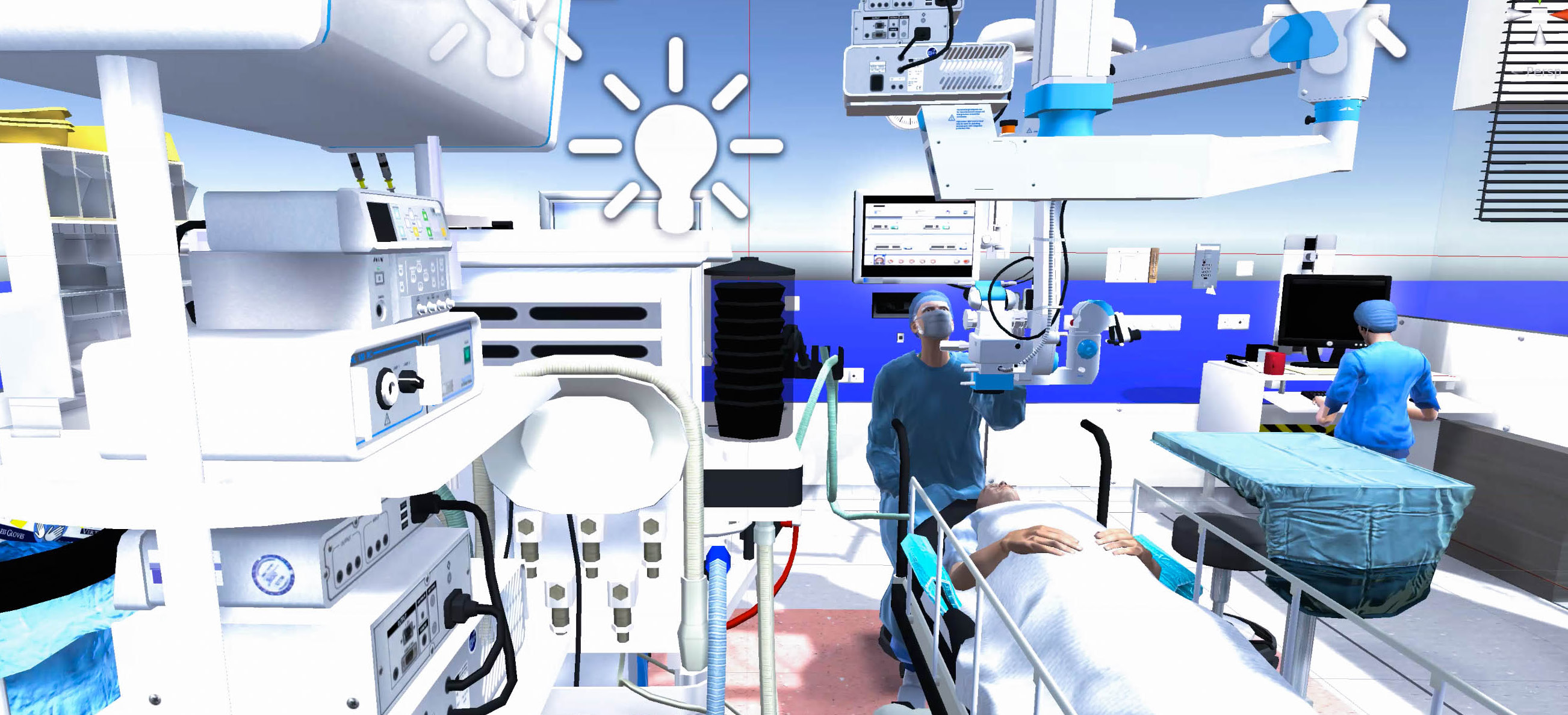
Mentoring Students in Social VR
The VRTogether project conducts ground-breaking research on Social VR. In addition, the project mentors master’s students in this area, forming a new generation of graduates that can, in the future, reshape the media landscape. CWI has been active, offering master’s thesis around the core topics of the project. Last year, two students graduated at TU Delft with the theses “Multi-Camera Registration for VR: A Flexible, Feature-based Approach” (Qian Qinzhuan) and “User Experience in Social Virtual Reality” (Yiping Kong). The latter resulting in a top quality paper, “Measuring and understanding photo sharing experiences in social Virtual Reality”, presented at ACM CHI 2019.
This year, again two students have graduated acquiring deep knowledge about the fundamentals of Social VR. Guo Chen (TU Delft) has written the thesis “Designing and Evaluating a Social VR Clinic for Knee Replacement Surgery” and Jelmer Mulder (VU Amsterdam) the thesis “Temporal Interpolation of Dynamic Point Clouds using Convolutional Neural Networks”. The first one explored novel use cases in the healthcare domain for Social VR, developing and evaluating a prototype of a Social VR clinic. Such exploration can help better understanding the exploitation opportunities. The second one proposed a temporal interpolation architecture capable of increasing, at the receiving side, the temporal resolution of dynamic point clouds. The results have consequences in the core architecture of the system, since it may allow for providing the same quality of experience of users, while using less bandwidth for delivering them.
Guo Chen successfully defended her master’s thesis, which explores how Social VR could be used in a hospital setting. The motivation behind this project was to support patients with limited physical mobility to travel fewer times to the hospital, but still communicate well with doctors and nurses. Patients with knee osteoarthritis were the target group of this work. The final goal was to build a Social VR clinic that simulates the real consultation room and facilities in the hospital, in which, patients can interact with the doctors or nurses with visualized information, such as surgery preparation procedures, 3D anatomy models and a tour in the surgery room. A series of ethnographic studies at the Reinier de Graaf hospital in Delft lead to a better understanding of the complete patient journey, and to the identification of the requirements for the design and prototyping of a Social VR solution. It supports the three main identified activities within the patent journey: visualization of the intervention process, “walking into” a 3D virtual surgery room to “meet” the medical staff and to get familiar with the equipment, and interacting with an animated virtual 3D knee anatomy model and a virtual knee prosthesis model to see what the differences are before and after the surgery. Twelve users were recruited to evaluate the Social VR clinic, who indicated that the Social VR clinic consultation is comparable with the face-to-face, but does not require patients with knee problems to go to the hospital.
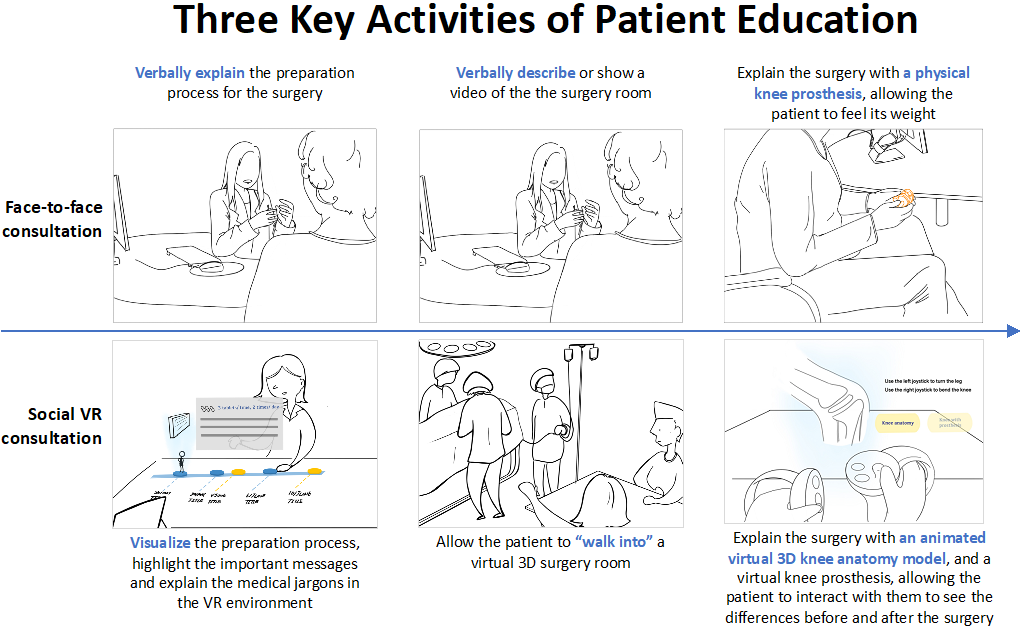

Jelmer Mulder as well successfully defended his master’s thesis, which explores how machine learning techniques can help in optimizing the distribution of dense point clouds. Point clouds are a data structure that models volumetric visual data as a set of individual points in space, which it is used in VRTogether for highly realistic representing users in a Social VR session. But point clouds are voluminous in size, and thus require high bandwidth to transmit. In practice this means that concessions have to be made either in spatial or temporal resolution. In his thesis he proposes an architecture capable of increasing the temporal resolution of dynamic point clouds. With this technique, dynamic point clouds can be transmitted in a lower temporal resolution, after which a higher temporal resolution can be obtained by performing the interpolation on the receiving side. The interpolation architecture works by first downsampling the point clouds to a lower spatial resolution, then estimating scene flow, and finally upsampling the result back to the original spatial resolution. To improve the smoothness of the interpolation result, a novel technique called neighbour snapping is applied, and in order to estimate the scene flow, a newly designed neural network architecture is used. The architecture was evaluated through objective metrics and based on a small-scale user study. Existing objective quality metrics for point clouds are known to have poor correlation with user perception and the findings confirm this: the metrics correlate poorly with themselves and with the results from the user study. The user study shows that on average, participants prefer the temporally interpolated sequences generated by our architecture over current state-of-the-art or sequences that have not been interpolated.
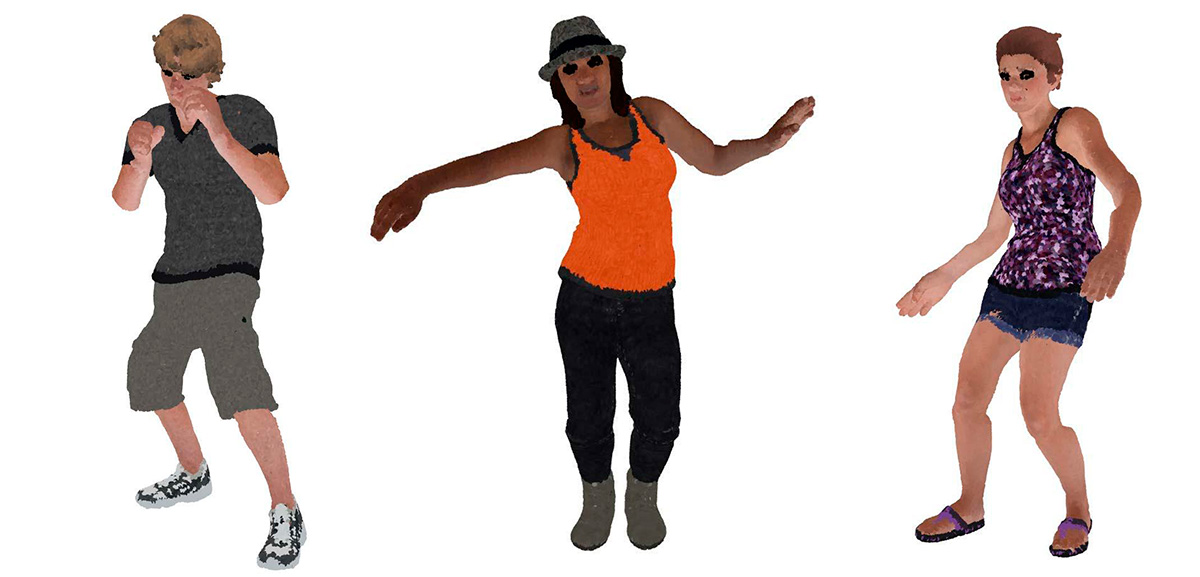
Congratulations to the recently graduated students!
Text: Jie Li and Pablo Cesar — CWI
Come and follow us in this VR journey with i2CAT, CWI, TNO, CERTH, Artanim, Viaccess-Orca, Entropy Studio and Motion Spell.

This project has been funded by the European Commission as part of the H2020 program, under the grant agreement 762111.